SPIn-NeRF: Multiview Segmentation and Perceptual Inpainting with Neural Radiance Fields
Mirzaei et al.
15 Mar 2023
A Novel Approach to Inpaint the NeRF Scene
While editing 2D images or 3D videos, one of the important tasks is to remove the undesired part (object) from the original scene. This task should be followed by replacing it with a region that is visually plausible and consistent with the background scene. When inpainting an object from a video, the edited 3D scene must maintain multiview consistency and geometric validity.
SPIn-NeRF introduces a novel approach that inpaints NeRF scenes, given a single-view object mask. Prior to the multiview inpainting method, it offers a multiview segmentation method to simplify the data (annotation) preprocessing. The segmentation method involves translating sparse pixel-level clicks into the segmentation of the undesired object, and then translating the target object into a 3D mask which can be interpreted by NeRF representation. Since the NeRF scene is composed of entangled, uninterpretable, and thus complex representations, the paper focuses on two perspectives in inpainting 3D NeRF scenes: (1) preserving fundamental 3D properties, and (2) expressing perceptually realistic appearance. To sum up, this paper provides a unified framework from multiview object segmentation to novel view synthesis of the inpainted NeRF scene, while enforcing the 3D viewpoint consistency.
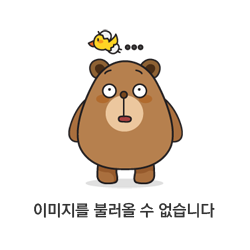
Multiview Segmentation
Existing approaches, which are based on interactive 2D segmentation methods, were not able to manage the 3D aspects of the problem. To resolve the 3D inconsistencies, the paper uses a semantic NeRF model (Instant-NGP as the architecture) for obtaining the multiview masks. It takes input RGB images, correspoding camera intrinsic/ extrinsic parameters, and initial masks to calculate both the classification and reconstruction loss so that the semantic NeRF can be trained.
Multiview Inpainting
SPIn-NeRF utilizes perceptual losses to account for inconsistencies in the 2D inpainted images, as well as inpainted depth images to regularize the geometry of the masked region. The paper insists that the perceptual loss obtained by the RGB priors does not return satisfiable results, hence leverages the outputs of 2D inpainters (e.g., LaMa) as appearance and geometry priors to calculate the depth loss.
Perceptual and Semantic Thinking
By using the semantic NeRF model, the AI can segment out the object by mere sparse annotation. Also, by using the perceptual loss, the AI inpaints the unwanted object from the 3D videos. It is somewhat afraid to inject the perception and semantics in the process of AI thinking.
'View Synthesis' 카테고리의 다른 글
NeRF: Exploring the Frontiers of Modern 3D Scene Reconstruction (0) | 2024.02.23 |
---|